If a test to detect a disease whose prevalence is 1/1000 has a false positive rate of 5%, what is the chance that a person found to have a positive result actually has the disease, assuming you know nothing about the person’s symptoms or signs?
We assume that the test correctly diagnoses patients who have the disease.
This question was asked to 60 Harvard Medical students in 1978 by Schoemberger, Grayboys and Casscells.
The average of student’s answers was 0.95… while the correct answer is 0.02.
What happened?
The base rate fallacy is an error, a cognitive “jump”, that occurs when the conditional probability of event A knowing B is assessed without taking into account the prior probability of B.
In this sense, this cognitive bias prevents us from following the Bayes Theorem.
As a reminder, most of us have studied probabilities at high school and should have heard about the below Theorem:
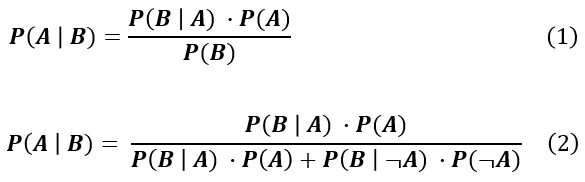
In our example, let’s say that:
D represents the event “The patient has the disease” and P(D) represents the probability that the patient has the disease. This probability is 1/1000.
D_ represents the event “The patient does not have the disease”. The probability is P(D_) is 0,999.
P represents the event “The test is positive”.
P(D/P) is the probability that the patient has the disease, knowing that the patient has a positive test. This is what we want to compute.
P(P/D) is the probability is that the test is positive, knowing that the patient has the disease. This probability is 1.
P(P/D_) is the probability that the test is positive knowing that the patient is not ill. This probability is 0.05.
What most people do:
They ignore the prior probability of disease prevalence. The test is wrong in 5% of cases. This means that if the test is positive, in 5% of cases, this is not true. Thus the probability to have a positive test and have really the disease is 95%.
In their head, P(D/P) is equivalent to 1-P(P/D_).
Correct answer (based on Bayes’ Theorem):
The probability of having the disease, knowing that the test is positive is
P(D/P)=P(D)*P(P/D)/P(P)= P(D)*P(P/D)/(P(D)*P(P/D)+ P(D_)*P(P/D_)))
=0,001*1/(0,001*1+0,05*0,999)
=2%
This bias affects a lot of our investment decisions. When we read the description of a fund, for instance, we tend to focus on immediate past performance to have an idea of the future performance, without taking into account that the available data is limited.
The base rate fallacy can also explain the survivorship bias, where we interprete the average good performance of funds without taking into account the results of those which have disappeared.
Reference
Gigerenzer, G. (1993). The bounded rationality of probabilistic mental models. In This chapter is based on a lecture delivered at Harvard University, Oct 2, 1991.. Taylor & Frances/Routledge.